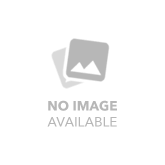
Deep learning in artificial intelligence
Deep learning in artificial intelligence is one of the most important innovations developed by modern technology, as it helps in developing intelligent systems that can recognize patterns and extract important information from huge data.
Deep learning AI is used in many fields, which makes it of great importance in our daily lives.
During this article, we will learn about everything related to deep learning in artificial intelligence, deep learning applications, deep learning strategies, and everything related to it.
What is the concept of deep learning in artificial intelligence?
Deep learning in artificial intelligence is a branch of artificial intelligence that relies on deep neural networks, which are networks that have several layers that can process complex data in a very advanced way, allowing machines to learn and analyze data like humans.
Why is deep learning in AI important?
The importance of deep learning in artificial intelligence comes from its ability to process and analyze complex data in very advanced ways, and this provides high performance and great accuracy in many applications. The most important reasons why deep learning is central to artificial intelligence include:
- Processing huge amounts of data: Recent developments in technology have led to the availability of huge and complex data, such as images, videos, text, and audio. Deep learning relies on deep neural networks, which can absorb this huge data and process it in more accurate ways than traditional models.
- Complex Pattern Recognition: Deep learning is characterized by its ability to extract complex features from raw data automatically, without the need for manual feature customization. The system can learn subtle patterns that would be hidden even to a human, making it effective in applications such as image recognition, voice analysis, and machine translation.
- Accuracy and flexibility in performance: Deep learning allows systems to learn and improve over time. When deep learning models are trained on sufficient amounts of data, they gain the ability to predict more accurately, which increases the systems' efficiency in performing various tasks including facial recognition, fraud detection, and medical data analysis.
- Advanced applications: Deep learning applications are the basis for several modern applications, such as self-driving cars, which rely on real-time image analysis and interaction with the surrounding environment, as well as in healthcare applications, where it is used to analyze medical images and early detection of diseases.
- Intelligent interaction and adaptation: Deep learning enables systems to interact more intelligently with users. For example, in smart assistant applications (such as Siri or Alexa), deep learning is used to analyze voice, provide accurate responses, and understand the context of speech.
In short, deep learning in artificial intelligence is a powerful tool that revolutionizes the ability to process and analyze data, making systems more efficient, intelligent, and able to adapt to different environments and provide more effective solutions in various fields.
How is deep learning used in modern applications?
The uses of deep learning in artificial intelligence are many and varied, as it has proven its effectiveness in many vital fields that require processing huge and complex data. Here's a breakdown of some of the main applications of deep learning:
Image recognition and computer vision:
Deep learning in artificial intelligence is the backbone of image recognition systems. Convolutional neural networks (CNNs) are used to analyze images and detect patterns in them, which makes them useful in many applications such as:
Facial recognition: Deep learning is used in security applications, such as surveillance cameras and security systems, to identify people.
Medical imaging: Deep learning models help analyze medical images such as X-rays and MRIs, which helps doctors detect diseases such as cancer and heart disease in their early stages.
Self-driving cars: These cars rely on computer vision to recognize objects and people, and understand the surrounding environment to make decisions in real time.
Voice analysis and speech recognition:
Deep learning in artificial intelligence is used in voice processing techniques to recognize and convert speech into text, and is a core technology in voice assistant systems such as Siri and Alexa.
It is also used in voice sentiment analysis, where models can analyze the tone of voice and understand the speaker's emotions, which is useful for improving user experiences in customer service and applications that interact with users.
Natural Language Processing (NLP):
Deep learning in AI can analyze and understand human language in advanced ways, and is widely used in:
Machine translation: such as Google Translate, where the model analyzes sentences and translates them into other languages thanks to advanced translation networks.
Text and sentiment analysis: Models can analyze the content of texts and identify the sentiments associated with them, which is used to evaluate user opinions on social media and analyze public reactions.
Smart conversations: The development of smart chatbots relies on natural language processing, as they can respond to user inquiries naturally and logically.
Forecasting and analysis in financial markets:
Deep learning models in artificial intelligence help predict the movement of financial markets by analyzing data on stocks, currencies, and economic indicators. These models can study historical patterns and interact with influencing factors to make more accurate investment decisions.
Cybersecurity:
Deep learning is used in artificial intelligence to detect fraud and cyberattacks by analyzing user behavior and threat patterns, and improves the protection of networks from malicious attacks. For example, intelligent systems can recognize unusual patterns that may indicate fraudulent attacks.
Healthcare and diagnostics:
In the health sector, deep learning in artificial intelligence is used to develop systems that help doctors diagnose and treat diseases. By analyzing patient records and medical data, models can make personalized recommendations and predict health outcomes, such as the risk of heart disease.
Smart robots:
Deep learning in artificial intelligence helps in developing smarter and more effective robots. These robots use neural networks to recognize objects and interact with the surrounding environment, allowing them to be used in industries to perform complex tasks accurately and efficiently, such as robots used in factories and stores.
Precision agriculture:
Deep learning in AI is used to improve agricultural productivity by analyzing satellite and drone images to learn about the condition of crops, which helps in making more accurate decisions about irrigation, fertilization and harvesting.
Games and artificial intelligence in entertainment:
In the gaming industry, deep learning in AI can create intelligent characters that interact dynamically with players. It is also used to create entertainment content, such as improving the quality of photos and videos and analyzing user behavior to provide personalized entertainment experiences.
Ecommerce and Personalization Recommendations
Deep learning in artificial intelligence relies on analyzing customer behavior in online stores to provide personalized recommendations. Such as the recommendation systems used by Amazon and Netflix, where movie or product recommendations are made based on the customer's preferences and history.
How does deep learning work in artificial intelligence?
Deep learning in AI works by training neural networks using huge sets of data, where the system automatically learns to extract features and analyze patterns to arrive at accurate results.
Components of deep learning networks in artificial intelligence
Deep learning networks in artificial intelligence consist of a set of layers that together contribute to building a model capable of processing and analyzing data in advanced ways. In deep learning networks, data is passed from one layer to another, and each layer performs special tasks that help extract the patterns and features required to reach accurate results. Here are the components of a deep learning network in detail